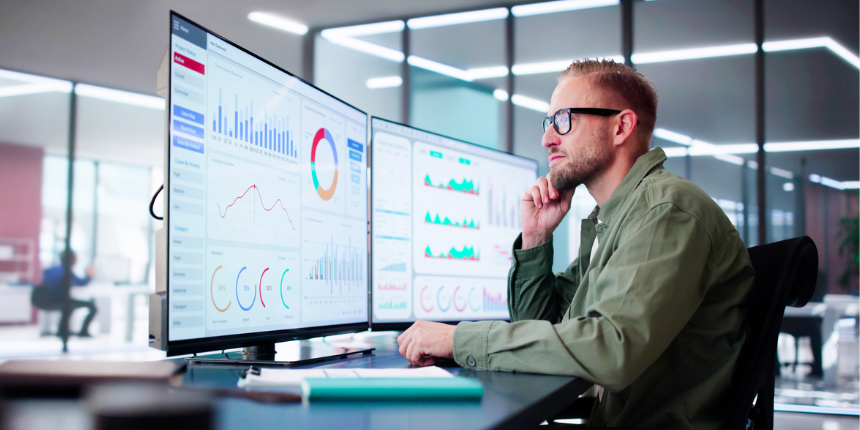
Unleash the true value of your data with our center of excellence, Proove Intelligence.
In the world of digital marketing, we no longer operate in isolation. As a brand, you’re likely investing across multiple channels—digital, out-of-home (OOH), TV, radio, and print—each influencing the consumer journey and competing for your marketing dollars. In short, understanding which channels drive sales is crucial.
From the days of single-channel campaigns to the multi-faceted digital ecosystems of 2024, marketers have continuously sought better ways to attribute conversions to their rightful sources. In this article, we’ll explore the evolution of attribution modeling, examining various models and how they’ve been changed to meet today’s digital demands for effective marketing strategies.
Attribution modeling is the process of assigning credit to the touchpoints a consumer encounters along their customer journey. Essentially, it’s about understanding which channels, campaigns, or specific actions contributed to the decision to buy and to what extent. The marketing mix now also includes channels such as social media, influencer partnerships, voice search, and augmented reality experiences, all of which require an updated approach to attribution.
Furthermore, the introduction of GDPR, CCPA, and other privacy-focused legislations has challenged and reshaped the way data is collected and analyzed, making the need for transparent and compliant attribution practices paramount. This period of change isn’t just about adapting to new privacy norms; it forces us to explore privacy-first attribution.
Imagine a consumer named Alex, who is in the market for a new smartphone. Alex’s journey to purchase might look like this:
In the era of heightened data privacy concerns, marketers have pivoted to privacy-first attribution models. These models prioritize the use of first-party data—information directly collected from your audience through interactions with your brand, such as website visits, app usage, or customer feedback. For instance, a retail brand might analyze data from its loyalty program to understand which marketing efforts are leading to repeat purchases, all while ensuring customer data remains protected.
Additionally, when third-party data is used, it’s anonymized to safeguard individual identities. This could involve aggregating data from social media interactions to identify trends without linking information to specific users. A practical example is a marketing team analyzing anonymized location data to see how outdoor billboard placements influence online shopping behavior in different regions, ensuring individual privacy is respected.
Subscribe to our monthly newsletter.
With the average consumer using multiple devices daily, cross-device attribution has become a cornerstone of modern marketing strategies. This approach leverages advanced tracking technologies, such as unified customer IDs and probabilistic modeling, to stitch together user interactions across smartphones, tablets, laptops, and more.
Imagine a scenario where a consumer sees a product ad on their smartphone, conducts research on a tablet, and makes a purchase on a laptop. Cross-device attribution enables marketers to track this journey seamlessly, attributing sales correctly across each touchpoint. For example, a tech company might use cross-device data to understand how YouTube reviews watched on mobile influence software purchases made on desktops, offering a cohesive view of the consumer journey.
Predictive analytics transforms historical data into forward-looking insights, allowing marketers to anticipate trends and adjust their strategies accordingly. This involves using machine learning algorithms to analyze past marketing campaigns, sales data, and customer interactions to predict future behaviors and preferences.
For example, a streaming service could analyze historical viewing patterns, subscription upgrades, and content preferences to predict which genres or titles will drive engagement and retention in the coming months. Similarly, a fashion retailer might use predictive analytics to forecast seasonal trends, enabling them to tailor their marketing campaigns to upcoming demands, optimizing both inventory and advertising spend.
By incorporating these advanced methodologies, marketers can navigate the complexities of the digital landscape more effectively, ensuring that their strategies are both privacy-compliant and adaptable to the ever-changing patterns of consumer behavior.
Attribution modeling can be applied to both online and offline activities and can also extend to things that are outside of your control.
Types of online attribution include your website, paid search and display campaigns, organic rankings in SERP’s, social media and content. Offline attribution encompasses traditional marketing mechanisms such as out-of-home (billboards, transit, street furniture and other), print (magazines, newspapers, flyers, and pamphlets), radio, and television.
But even the most perfectly crafted and symbiotic marketing strategy does not guarantee results. Brands need to remain acutely aware of non-marketing factors that could simultaneously be at play eroding the potential of your marketing initiatives. Variables such as the economy, politics, cultural tastes and biases, geography and even weather can all disrupt marketing campaigns. A failure to keep a pulse on these factors can render any marketing efforts moot even before they are deployed.
For example, you have this great new product that you want to promote. You work with your agencies to develop the right messaging, to the right audience, in the right places and at the right times. But after the first month, there has been no traction and eyebrows are starting to raise. What could have possibly gone wrong?
Upon further examination, you realize that the state with the highest concentration of your target audience recently experienced a huge downturn in the economy. Regardless of the value your product offers pockets are tight and disposable income is at a minimum. Until the economy improves, or unless you start giving your product away for free, the conditions just aren’t there to achieve the projected results.
While diving into attribution modeling might seem daunting, the insights it provides are invaluable for optimizing your marketing strategy and outperforming your competition. Start experimenting with different models and use the insights they produce to tweak your marketing investments to ensure the highest ROI and an advantage over your competition.
Your customers shed data. Every click, every call, every interaction with your brand leaves signals, clues, and sometimes even step-by-step guides on how better to serve and delight them. Your job is to listen and respond. Our job is to help with data analytics. Proove Intelligence by DAC is our multidisciplinary team of Data Engineers, Analysts, Math Geeks that specialize in attribution. They work closely with experts in Strategy, Content, Creative, and Media to turn data analytics into a potent force for transformational growth.
Unleash the true value of your data with our center of excellence, Proove Intelligence.
Unleash the true value of your data with our center of excellence, Proove Intelligence.
Unleash the true value of your data with our center of excellence, Proove Intelligence.
Subscribe to our monthly newsletter.