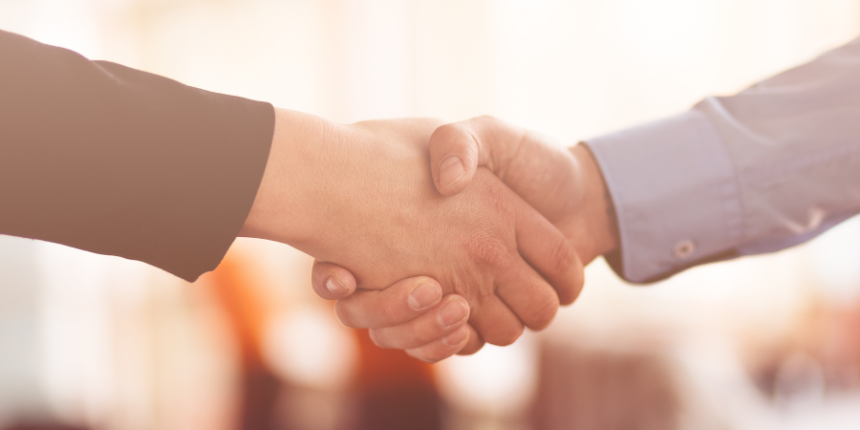
Navigate an unpredictable landscape with actionable, data-driven strategies tailored for your business from the brand down to the local level.
Maximising the basket value. It’s the Holy Grail for any e-commerce website. But what’s the best way to upsell at the basket? Why do people impulse buy? Why do different groups of people add products of different value? In this week’s analytics roundup, I’ll help answer these key questions with the help of analytics data. The research was based on the data of a large multi-channel retailer operating both online and offline.
Summary On average, customers are willing to add an extra (impulse) purchase to their shopping basket if the price of the additional product does not exceed 20% of the main product. However, there are some differences in impulse purchase behaviour between low and high spenders.
The idea The main idea of the research was rooted in the psychology of decision-making. It simply says that each potential customer assesses their gains and losses in relation to their main reference point and not the actual value of the purchase. It’s worth mentioning that reference points are very different for different people and change dynamically over time. For example, decision-making around Christmas is mostly driven by emotions and feelings with few people able to resist the mass hysteria of the Christmas season. So, the main reference point “how much to spend on a Christmas present to your auntie” is highly dependent on the nature of your relationship with her. Therefore, impulse sales for online and offline retailers, hospitality services, airlines, and anything else which could be marketed as “treating yourself” or “treat a loved one” peak around Christmas. The question is: How can you stimulate impulse purchases while leaving your customers delighted with their purchases despite the extra spend? The answer is in that simple concept illustrated above, the excitingly named Prospect theory value function developed by Kahneman (Nobel Prize in Economics, 2002) and Tversky in 1979. Despite the age of the concept it remains relevant even now, particularly when it comes to online shopping. Some data mining is essential to identify the patterns in customer purchase behaviour in order to increase the likelihood of additional impulse shopping.
Main research hypothesis In this research I assumed that: For users who already have some planned purchases, the main reference point would be the first product visitors demonstrate a significant interest in, or, even more reliably, the first product the add to their shopping cart. In both cases the value of a product can be tracked with Enhanced e-commerce in Universal Analytics. You should find the right balance between the value of planned and impulse purchases so the price of the “impulse” purchase (suggested as a recommended product) doesn’t exceed a certain percentage of the price of the main product in the cart. Let me illustrate this with an example. When a customer intends to buy a computer for £1,000, spending an additional £50 on speakers seems insignificant. However, a standalone purchase of speakers for £50 can feel quite different and considered with more caution. Thus, spending more seems insignificant only relative to the price of overall basket value. Following this, when consumers aggregate spending, diminishing sensitivity makes it much less painful to spend additional funds in the presence of more expensive items. Customers who buy relatively expensive products should be more exposed to impulse shopping if an extra product is cheaper and can be related to the customer’s needs. So, low, medium and high price of the main products would define the limit on the price for impulse purchases.
Data
Subscribe to our monthly newsletter.
Stay Forward
Results During this research the correlation between the prices of supplemented products and main products in the shopping cart showed that customers willingly added some impulse purchases to their shopping cart if those supplemented products did not exceed 20% of the most expensive item in the shopping cart. Low spenders (less than £50) do not have any special patterns in their impulse purchases and their impulse products can be more expensive than the main product they intended to buy initially. Please see the distribution of price ranges on the additional products in comparison to the main purchases below.
Application for business Tweaking the algorithms which display recommended products in e-commerce for higher efficiency is an area of research that keeps thousands of data scientists busy around the globe. A possible application of these research results would be placing a special plugin containing a random choice of products or services, which are likely to be bought on impulse, on the website’s shopping cart. Also, it might be beneficial to add this plugin to other product pages and show to a customer after there is at least one item in customer’s shopping cart. Data on what items were added, when, and their overall value, can generate useful insights for any e-commerce business. However, each business is unique and the results of similar research could vary for different companies / product categories / target audiences. I would recommend making your initial data mining research on customer impulse purchase patterns and only then to apply those customised results in practice. Otherwise you are taking action without insight, which is always a dangerous move!
Want to see how we use data visualisation make bespoke dashboards to demonstrate how each channel attributes to your business goals (on line and offline) ?
Read an overview of our Marketing Analytics Services
Got a question about analytics? Ask Yana now.
Navigate an unpredictable landscape with actionable, data-driven strategies tailored for your business from the brand down to the local level.
Navigate an unpredictable landscape with actionable, data-driven strategies tailored for your business from the brand down to the local level.
Navigate an unpredictable landscape with actionable, data-driven strategies tailored for your business from the brand down to the local level.
Subscribe to our monthly newsletter.